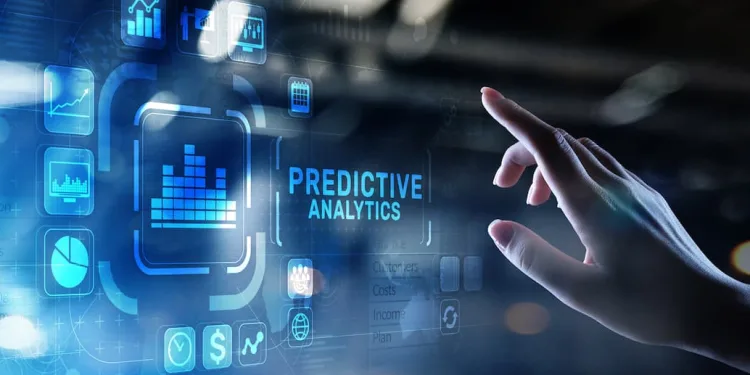
Leveraging Predictive Analytics For Advanced Audience Targeting
Predictive analytics is an evolving field that offers businesses an unprecedented view into potential future events based on historical data. It moves beyond just understanding what has happened to provide insights into what might happen next.
How Does It Work?
Basics: At its core, predictive analytics employs statistical algorithms and machine learning techniques on historical data to identify patterns. By understanding these patterns, businesses can make educated predictions about future events.
Applications: Imagine an e-commerce company trying to anticipate their sales for the holiday season. Using predictive analytics, they can analyze previous years’ sales data, current market trends, and even social media sentiment to estimate their sales. Financial institutions often use it to assess the likelihood of loan defaults or to recognize potentially fraudulent activities by identifying transactions that deviate from a user’s typical behavior.
Implementing Predictive Analytics:
Objective Definition: Clearly state what you’re trying to predict. This can range from understanding customer churn rates to forecasting inventory needs.
Data Gathering: Collate all relevant historical data. The richness and accuracy of this data can significantly impact prediction quality.
Model Selection: Depending on the nature of your data and what you’re trying to predict, choose a suitable model. Tools like Scikit-learn in Python or R’s vast library can be invaluable.
Model Training: Feed your data into the model, allowing it to learn from past trends.
Refinement: Continually improve the model by feeding it new data, tweaking its parameters, and validating its predictions.
Traditional vs. Predictive Analytics:
While traditional analytics might tell a retailer how much of a particular product they sold last month, predictive analytics might suggest how much of that product they’ll sell next month. Traditional analytics helps businesses understand past performance, but predictive analytics provides a roadmap for future actions.
Customer Segmentation:
Definition: Customer segmentation involves categorizing your customer base into distinct groups based on shared characteristics, which can range from demographics to behavioral patterns.
Deep Dive:
- Behavior-Based Segmentation: This approach looks at past interactions customers have had with your business. This could include purchase history, website interaction, response to past marketing campaigns, etc.
- Demographic-Based Segmentation: Here, customers are grouped by age, gender, income, education, etc. For instance, a luxury brand might target a segment with a higher income bracket.
- Predictive Aspect: Once segments are defined, predictive models can be used to determine the likely future actions of these groups. For instance, if a segment historically shows high engagement during holiday sales, marketing efforts can be ramped up for this group during such periods.
Benefits: Understanding these segments allows businesses to tailor marketing messages, offers, and products to specific groups, making promotional efforts more effective and efficient.
Product Recommendations:
Definition: This involves suggesting products to users based on their past behavior, preferences, or other relevant metrics.
Deep Dive:
- Collaborative Filtering: This method recommends products based on what similar users have liked. For instance, if User A and User B both liked Product X, and User A liked Product Y, then Product Y might be recommended to User B.
- Content-Based Filtering: This is based on the attributes of products and a profile of the user’s preferences. For example, if a user frequently purchases thriller novels, they will receive recommendations for other thrillers.
- Predictive Aspect: By analyzing vast amounts of data, predictive models can accurately forecast which products a user is likely to be interested in next.
Benefits: Enhances the user shopping experience, increases average order values, and boosts sales.
Churn Prediction:
Definition: Churn prediction aims to identify which customers are most likely to stop using a product or service.
Deep Dive:
- Indicators: A decrease in usage frequency, negative feedback, or reduced engagement with marketing materials might signal a customer’s intent to churn.
- Predictive Aspect: Predictive models analyze past customer behavior and other factors to predict which customers are most at risk of churning.
Benefits: Proactively addressing potential churn can lead to higher retention rates, sustained revenue, and improved customer relationships.
Dynamic Pricing:
Definition: Dynamic pricing involves adjusting prices in real-time based on current market demands.
Deep Dive:
- Factors Considered: Real-time data, such as current demand, competitor pricing, and other external events, are taken into account.
- Predictive Aspect: Predictive analytics can forecast demand surges or drops based on historical data, external events, and more, enabling businesses to adjust prices preemptively.
Benefits: Optimized pricing can maximize profits, ensure competitive pricing, and better match supply with demand.
Content Personalization:
- Definition: It involves tailoring content to individual user preferences and behaviors to increase engagement.
- Deep Dive:
- Viewing Habits: Platforms like Netflix analyze user viewing habits to suggest content they might enjoy.
- Predictive Aspect: By understanding a user’s past interactions, algorithms can predict future content preferences, even introducing users to new genres or shows they haven’t explored yet.
- Benefits: Personalized content increases user engagement, prolongs platform usage, and enhances user satisfaction.
Refining Marketing Efforts:
Deep Dive:
- Historical Data: Analyzing past campaigns can provide insights into what worked and what didn’t. Which demographic responded best to which ad? Which marketing channels yielded the highest ROI? Answers to these questions can refine future strategies.
- Predictive Aspect: By examining the patterns in past interactions, predictive models can forecast which segments are more likely to engage with a particular campaign.
Marketing Implementation:
- A/B Testing: Implement A/B tests to validate predictions. For instance, if the model suggests a particular segment prefers video content, test video ads against image ads for this segment.
- Tailored Content: Customize marketing messages for identified segments. If predictive analytics indicate that a segment prefers eco-friendly products, then tailor your content to highlight the sustainable aspects of your products for that segment.
Seasonal Strategy:
Deep Dive:
- Past Trends: By analyzing sales or engagement data from previous years, businesses can identify seasonal patterns. For instance, there might be a spike in certain product sales during the holiday season.
- Predictive Aspect: By recognizing these patterns, businesses can predict demand for products or services during specific times of the year.
Marketing Implementation:
- Inventory and Promotion: If predictions indicate a surge in demand for a product during a particular season, ensure adequate inventory and run promotional campaigns leading up to that period.
- Timely Content Calendar: Develop a content calendar aligned with seasonal insights. If your audience engages more with summer-related content in June, schedule relevant campaigns, blogs, or social media posts during that time.
Challenges and Considerations:
Data Privacy:
Deep Dive:
- Regulations: Many regions have strict data privacy laws, such as the GDPR in Europe. Compliance is not just a legal necessity; it’s also vital for maintaining customer trust.
Marketing Implementation:
- Transparency: Clearly communicate to your audience how their data is used. Offer easy opt-in and opt-out options.
- Stay Updated: Regularly update the team on changing data privacy laws. Conduct periodic training sessions to ensure everyone understands and adheres to them.
Data Quality:
Deep Dive:
- Consistency: Irregular or missing data can skew predictions. It’s vital to maintain a consistent data collection process.
- Audits: Regular data audits can identify and rectify inaccuracies.
Marketing Implementation:
- Feedback Loop: Create a feedback mechanism where the marketing team can report data discrepancies. For instance, if they notice that campaign engagement data is not syncing properly with the analytics dashboard.
- Collaboration: Work closely with the IT or data team to ensure that the data being used for marketing predictions is of high quality.
Scalability:
Deep Dive:
- Infrastructure: As the volume of data grows, the infrastructure (like servers and databases) should support this growth without compromising performance.
- Model Complexity: With growth, businesses may want to incorporate more variables into their predictive models, which will require more computational power.
Marketing Implementation:
- Future-Proof Strategies: Develop marketing strategies that are adaptable to change. As the business scales, campaigns or strategies might need to pivot quickly based on new predictive insights.
- Regular Sync with IT: Regularly check with the IT department to ensure that the analytics tools being used by the marketing team are scalable and can handle the increasing complexity of predictive tasks.
Predictive analytics in audience targeting is a transformative force. It’s not just about crunching numbers; it’s about understanding your audience deeply and crafting resonant experiences. As the digital landscape continues to evolve, businesses must harness this power to stay ahead. It’s time to let data-driven insights steer the ship.